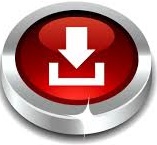
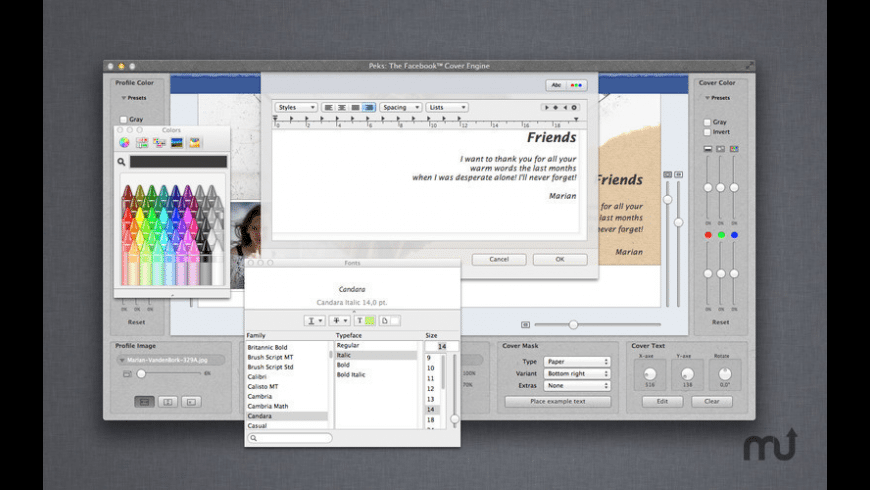
2015 Meinshausen and Bühlmann 2010 Shickel et al. The tool, named Wordify, uses an approach to supervised machine learning known as randomized logistic regression ( Johannsen et al.
#Mac wordify free#
The purpose of this article is to address this gap by introducing and illustrating a new, free online tool that allows researchers who lack advanced training in natural language processing to perform such discriminatory vocabulary analyses. 2020), or how situational context affects the vocabularies that consumers use to express emotions of negative versus positive valence ( Jurafsky et al.
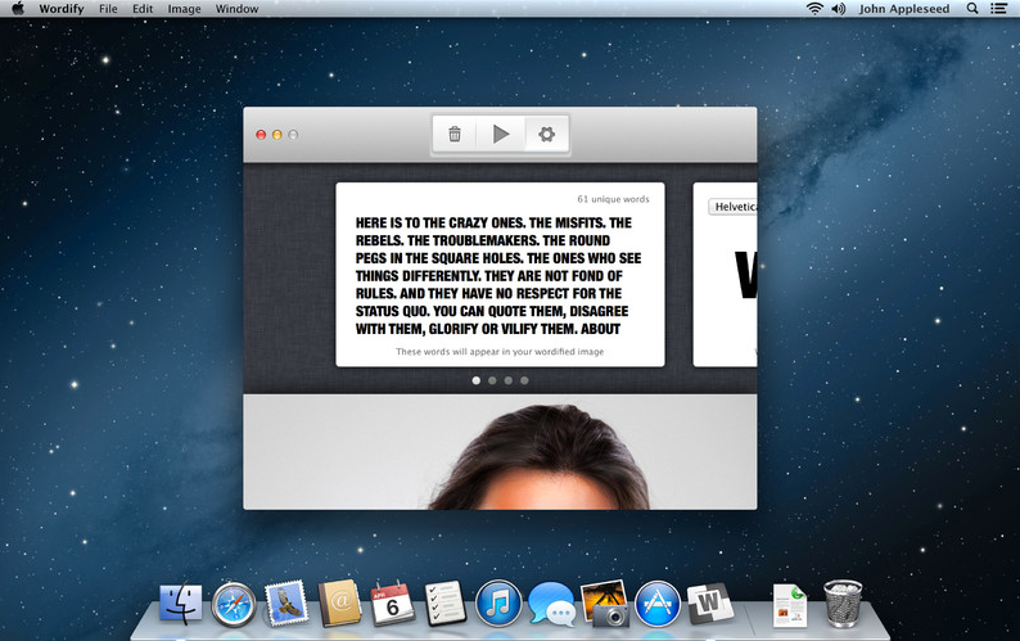
These tools include those that help identify the unique words that men versus women use when discussing the same topic ( Johannsen, Hovy, and Søgaard 2015 Joshi et al. While these methods use individual words as their primary inputs, fewer tools within consumer research have been developed wherein words serve as outputs. 2019) or the sentiment being conveyed ( Hartmann et al. 2019 Humphreys and Wang 2018 for recent reviews), most text-analysis tools seek to derive measures of the high-level features of texts, such as the topics being discussed ( Tirunillai and Tellis 2012 Toubia et al. While this literature is diverse in goal and method (e.g., see Berger et al. Paralleling the increased interest in the study of word use has been the development of automated text-analysis tools designed to facilitate such analyses. This topic has also gained traction in consumer health care, where gaps in doctors’ and patients’ vocabularies when discussing illnesses are widely seen as a major barrier to treatment ( Koch-Weser, Rudd, and DeJong 2010). For example, research has examined how changes in the use of pronouns (e.g., “we,” “you,” or “I”) can alter consumers’ views toward service providers and brands ( Packard, Moore, and McFerran 2018 Sela, Wheeler, and Sarial-Abi 2012), and how the inclusion of foreign words in ads can increase their persuasiveness among bilingual consumers ( Luna and Peracchio 2005). While work in this area varies widely in its goals, one focus of interest is how consumers choose specific words to convey ideas, and how those word choices affect behavioral outcomes. 2019 Humphreys and Wang 2018 Krishna and Ahluwalia 2008 Puntoni, de Langhe, and van Osselaer 2009). The study of how consumers use words to express thoughts, feelings, and preferences is a topic of growing interest among consumer researchers ( Berger et al. Text analysis, natural language processing, language, sentiment analysis A discussion is also provided on the use of Wordify in conjunction with other text-analysis tools, such as probabilistic topic modeling and sentiment analysis, to gain more profound knowledge of the role of language in consumer behavior. We show empirically that Wordify’s RLR algorithm performs better at discriminating vocabularies than support vector machines and chi-square selectors, while offering significant advantages in computing time. We present illustrative examples to show how the tool can be used for such diverse purposes as (1) uncovering the distinctive vocabularies that consumers use when writing reviews on smartphones versus PCs, (2) discovering how the words used in Tweets differ between presumed supporters and opponents of a controversial ad, and (3) expanding the dictionaries of dictionary-based sentiment-measurement tools. The tool, Wordify, uses randomized logistic regression (RLR) to identify the words that best discriminate texts drawn from different pre-classified corpora, such as posts written by men versus women, or texts containing mostly negative versus positive valence.
#Mac wordify install#
You can run ghc-pkg vector and if it doesn't say something like vector-0.9.1 then you need to run cabal update followed by cabal install vector.This work describes and illustrates a free and easy-to-use online text-analysis tool for understanding how consumer word use varies across contexts. Note the fixed indents and LANGUAGE pragma in the beginning to indicate that you use !lenTeens = VU.fromList - first element is "ten" 3 !lenOnes = VU.fromList - "", "one","two". | n < 20 = lenTeens `VG.unsafeIndex` (n-10) + pad | n < 10 = lenOnes `VG.unsafeIndex` n + pad

Import GHC.Base (Int(.), quotInt#, remInt#) What are the other files that I need to use for vectors? The compiler it said not found and asked me to use -v but this also gives an error. This program is about counting words but it gives an error in the header and I couldn't fix it.
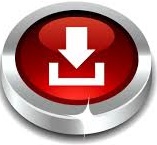